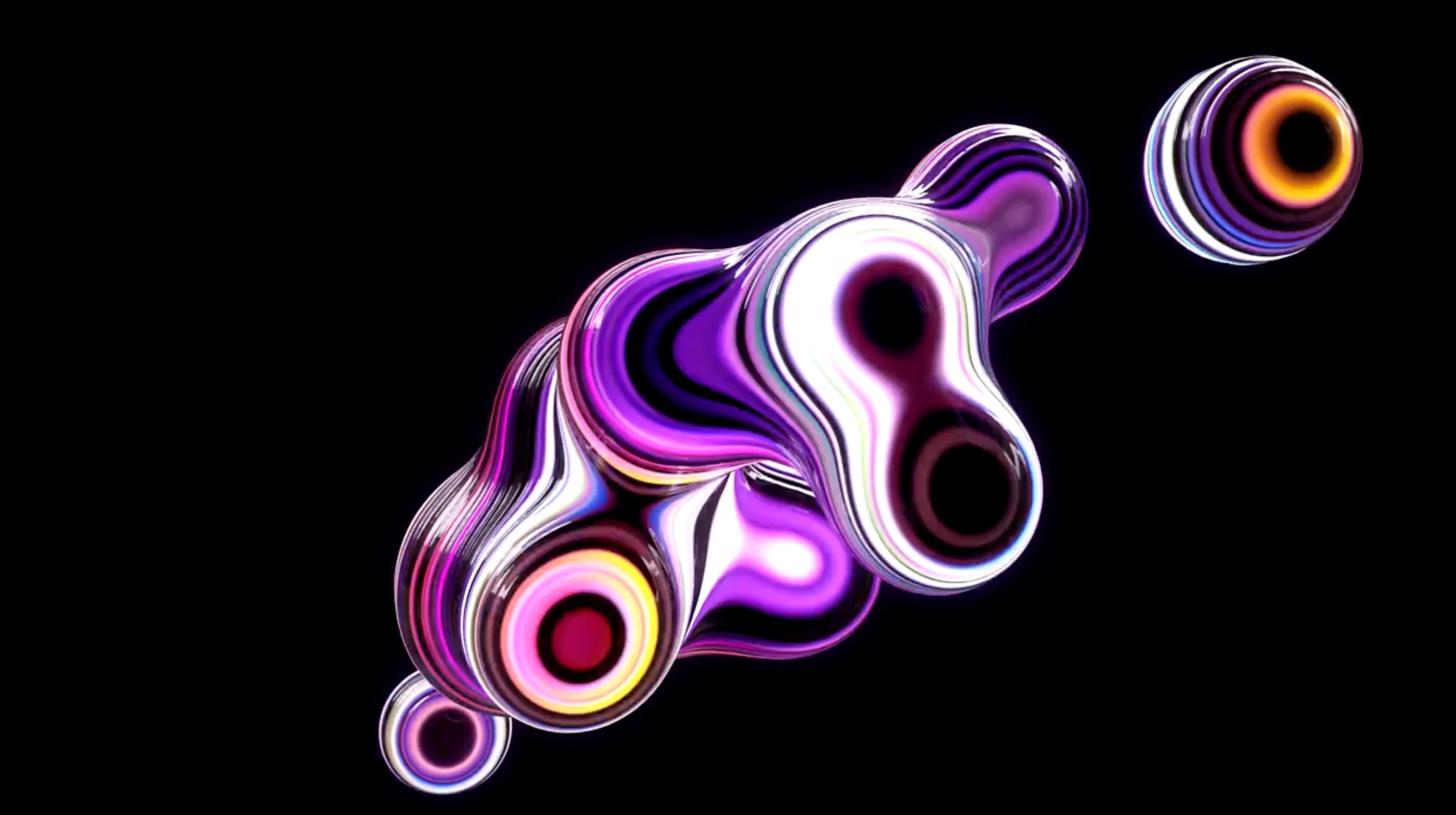
By Tom Khabaza, Principal AI Consultant at Solidatus
Earlier this year, we kicked off a blog series on active metadata. In that first blog post, entitled From data to metadata to active metadata, I provided an overview of what it is and the challenges it addresses.
To recap, metadata is data about an organization’s data and systems, about its business structures, and about the relationships between them. How can such data be active? It is active when it is used to produce insight or recommendations in an automated fashion. Below I’ll use a very simple example to demonstrate the automation of insight with active metadata.
In this – the second of this ongoing four-part series – we expand on the subject to look at the role of metadata in organizations, and how dynamic visualization and inference across metadata are essential to provide an up-to-date picture of the business. This is active metadata, and it is fundamental to the well-being of organizations.
Let’s start with a look at metadata in the context of an organization.
An organization and its metadata
Imagine a simple commercial organization. It has operational departments for sales, production and other operations, and corresponding systems which record their function. Transactions from each of these systems are copied into an accounting system, and from there into a financial report. The systems and the flow of data between them are depicted in a metadata model below:

Metadata showing systems and information flow
Data quality is also metadata
Financial reports must be accurate, both because they are used by executives in decision-making, and because they are part of the public face of the company, enabling it to attract investment and comply with regulations. The quality of the data in all the systems which feed these reports are therefore monitored closely, in this example using a data quality metric “DQ”, a property of each metadata entity, is scored from 1 (very poor quality) to 10 (top quality). In the metadata view below, these DQ values are shown as tags; in this example all the data is of top quality (DQ 10). Assuming that data quality measurement is automatic, these values will be updated automatically on a regular basis.

Metrics showing top-quality data
Data quality flows through the organization
A data quality metric is calculated from the data in the system to which it applies, but such metrics will often assume that the data being fed into the system is correct. This means that upstream data quality problems can have an undetectable impact on downstream data quality, so it’s important for data quality monitoring to take account of data flowing between systems. The metadata view below indicates the flow of good-quality data by a green arrow, and the presence of good-quality data by a green box. Again, this view indicates that top-quality data is present throughout. Unlike the previous view, however, constructing this view requires inference across the metadata: an entity will be displayed as green not only if it contains top-quality data but also only if all its incoming connections are providing top-quality data. This is a more informative view that will show the propagation of data quality problems when they occur.

Every data flow is top-quality
Spotting local data quality problems
Now suppose that a data quality problem occurs in the operational systems: the data quality metric is reduced from 10 to 9. The data quality metrics view shows the problem clearly but does not show its consequences; local data quality metrics imply that everything is all right downstream from the problem.

A data quality problem shows up locally
Inferring data quality problems actively
However, the more dynamic view of data quality shows the problem immediately. The active, inferential nature of this metadata allows us to color data flows red if they may be carrying low-quality data, and the systems red if they may hold low-quality data as a result of upstream data quality problems.

Active metadata inference shows the flow of data quality problems
The downstream transmission of data quality problems is obvious in this view: the financial report cannot be trusted. This dynamic alert is the first step towards fixing the problem, but it goes further than that. An alert system of this kind means that, when no alert appears, the data and reports are trustworthy, an important attribute of data systems both for management decision-making and for regulatory compliance.
Active metadata, inference and dynamic insight
To sum up, it is important that an organization recognizes metadata to be dynamic in nature and that this is made easy to visualize and react accordingly. It’s not enough to hold static information about the qualities of our systems and data; we must also keep it up to date with automatic metadata-feeds, infer continuously the consequences of any changes, and make these consequences visible immediately. Here I have illustrated the consequences of this approach for data quality and trust in financial reporting, but the same principles apply in a wide variety of contexts.
Wherever data is used – which today is almost everywhere – active, dynamic, inferential metadata makes it more informative, trustworthy, controllable and, ultimately, governable. Active, inferential, visual metadata is essential for the well-governed organization.
Quick Answer: What Is Active Metadata?
In the latest Gartner® report, find out what active metadata is, how to use it, and how to get started.